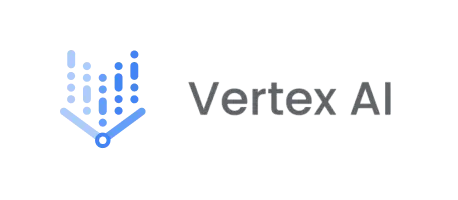
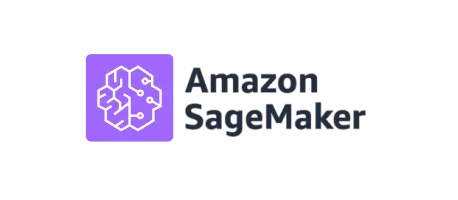
Feature | ![]() | ![]() |
---|---|---|
Zero-config model build & deploy | X | X |
Data source integration | GCP | AWS data sources |
Multi-cloud support | GCP | AWS |
Intuitive UI | X | X |
Support | Standard GCP support | Standard AWS support |
While offering a robust set of features, Vertex AI has a steeper learning curve, especially for those not already familiar with Google Cloud Platform. The platform is feature-rich but may require navigating through various services and configurations.
SageMaker, though powerful, demands a solid grasp of AWS and engineering expertise. Its UI is less intuitive than specialized platforms, requiring navigation and expertise through multiple AWS services.
Feature | ![]() | ![]() |
---|---|---|
Model build system | X | X |
Model deployment & serving | V | V |
Real-time model endpoints | V | Engineers required |
Model auto scaling | V | Engineers required |
Model A/B deployments | Engineers required | Engineers required |
Inference analytics | Engineers required | Engineers required |
Managed notebooks | V | V |
Automatic model retraining | Engineers required | Engineers required |
Using Vertex AI in production demands a broad skill set, including ML engineering, containerization, Kubernetes orchestration, Infrastructure as Code (with tools like Terraform or Google Cloud Deployment Manager), and networking (VPC, firewall rules). Additional GCP services like Google Cloud Storage, Google Kubernetes Engine (GKE), and Google Cloud Monitoring add complexity, requiring diverse engineering skills for effective management.
SageMaker does not have Training Jobs or simple deployment, and its Experiments feature and Studio IDE introduce complexity. The deployment and monitoring processes entail manual engineering setup, with limited out-of-the-box support.
Feature | ![]() | ![]() |
---|---|---|
Managed feature store | V | V |
Vector database | V | V |
Batch features | V | Engineers required |
Realtime features | V | Engineers required |
Streaming features | Engineers required | Engineers required |
Streaming aggregation features | Engineers required | X |
Online and offline store auto sync | X | X |
Vertex AI is partially managed, meaning some services are fully managed while others may require manual setup. For example, AutoML is fully managed, but custom training and data pipelines might require additional configurations or integration with other GCP services.
The AWS Sagemaker Feature Store requires manual setup for feature processes and lacks support for streaming aggregations, necessitating additional services like Elasticsearch, Chorma, Pinecone, and others for similar functionality.
Don’t just take our word for it
Qwak was brought onboard to enhance Lightricks' existing machine learning operations. Their MLOps was originally concentrated around image analysis with a focus on enabling fast delivery of complex tabular models.
Read Case Study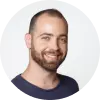
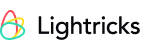