How Successful FinTech Startups Disrupt the Finance World with ML
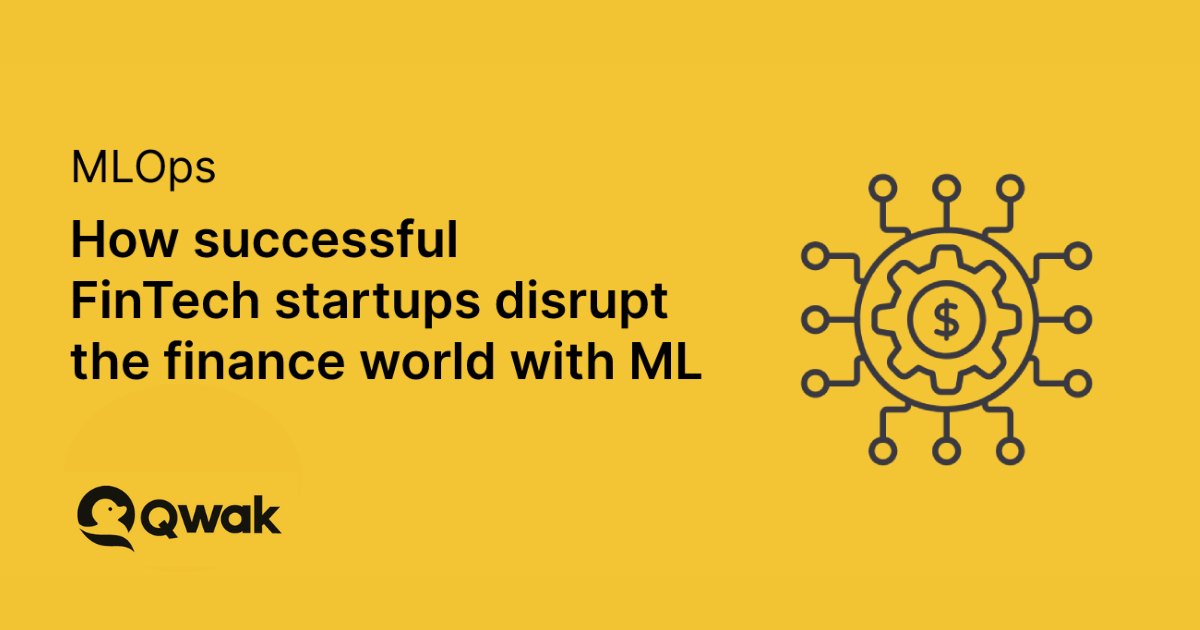
Decisions based on artificial intelligence and machine learning are an integral part of running a business in the data era. Machine learning helps businesses to serve customers quickly, and take decisions that directly contribute to the bottom line. It helps companies to detect fraudulent events and even prevent customers from defecting by providing irresistible offers at the right time. One factor that affects the successful usage of machine learning in a domain is the availability of data. Models learn based on the data they are trained on and the more data they have access to, the more useful they become.
Fintech refers to firms that use technology to optimize the delivery of financial services. It is one domain where a large amount of data gets accumulated. The majority of this data comes from transactional events. Fintech also benefits from behavioral data gathered from third-party sources because transactions are driven by behavioral aspects. The fintech domain also deals with many regulatory and compliance mandates and keeps data to adhere to the norms. It is safe to say that this is one of the most data-heavy industry domains.
Such an amount of accumulated data makes Fintech a hot favorite domain for machine learning-based reimagination. Another aspect that makes fintech a great use case for machine learning is the emphasis on quick decisions. Time is money and a second saved while making a decision is highly valuable in a domain that is based on money management. There are thousands of fintech startups that burn the midnight oil trying to save money or time by using machine learning on a large amount of accumulated data. A very small fraction of them become successful. Rest perish not being able to cope with ruthless decision makers of the financial world. This article will talk about how successful Fintech startups are disrupting the finance world with ML.
Understanding ML in finance
Artificial intelligence is a field of computer science that deals with mimicking human intelligence to solve complex problems. Engineers use rule engines, custom algorithms, logic, machine learning models, etc to implement artificial intelligence-based systems. Machine learning is a subset of artificial intelligence that uses data to bring human-level intelligence to software systems. Machine learning uses mathematical methods to arrive at equations that can approximate human decisions on historical data. The combination of equations and the weights associated with the variables in the equation is known as a model. Machine learning algorithms can either statistical ones or deep learning ones. Statistical machine learning relies on single iteration mathematical models while deep learning relies on a network of neurons where each neuron represents an equation and its weights.
Modern financial service organizations rely on machine learning to automate many tasks that needed human intelligence. Commercial banks, Investment banks, stock trading agencies, brokerage firms, insurance organizations, payment applications, etc are examples of businesses that benefit greatly from machine learning.
A commercial bank has numerous processes where a human being with financial knowledge and logical thinking needs to make decisions. For example, when an individual comes to a branch for a loan, how can the bank decide whether it is a good investment or not? The bank official asks for documentation about proof of the customer's income, his account statements, his previous history of loans, his medical history, and even his family details to make this decision. The process is even more complicated when the customer is another organization. The bank official then has to go through financial statements, the viability of the business, and numerous other factors before approving the loan.
Loans are not the only business that banks do. They invest in avenues like real estate, stock market, etc to make a profit out of the deposits that they receive from customers. At any point in time, the bank should maintain enough liquidity so that they don’t run out of cash when customers ask for their money back. How can the bank decide, the proportion of money that they should withhold from investing? Again, a senior employee who has studied the market for a long time makes this decision, also considering the regulatory requirements.
The Investment banks provide services related to investments and meeting capital requirements. They facilitate financing capital based on profit sharing, private equity investments, IPOs, etc. The employees in these organizations possess acute financial and business knowledge to identify sound investments. They project revenue and returns over decades and try to identify the sentiment of the public investors to arrive at prices during initial public offerings. All these tasks need human logical thinking and deep knowledge of the financial world and behavioral aspects of the market. Machine learning can help here by building models using years of data about decisions made by human beings in similar situations.
Insurance companies sell a guarantee of returning the money for loss of property or life by charging a monthly or yearly fee called the premium. The core success factor of running a successful insurance company is to arrive at premiums that provide enough cushion for the company to pay back and be appealing to customers at the same time. Insurance is a field with cut-throat competition and customers are always hunting for plans that offer the highest insured value and lowest premium. Insurance companies rely on years of experience of their employees to arrive at the premium and insured value. When employees make these decisions under pressure to meet their sales targets, naturally there are chances for errors. Machine learning can rely on years of data about these product configurations, how people reacted to them, and the money it brought the company to arrive at models.
Brokerage firms facilitate transactions and consume the difference between buying and sell values as their profit. Employees of brokerage firms are skilled enough to understand the demand and supply situation to great depths. They use this knowledge to set buying and selling prices to make both parties happy while leaving enough for their profit.
What we described here is only the operational aspect of running a financial business. Customer service is another area where machine learning can make a big difference in the fintech domain. Minimizing the interaction between employees and customers is a great way to reduce customer complaints and bring repeatability and reliability.
Disrupting the finance world with AI/ML
Successful startups use machine learning to disrupt the finance world by optimizing Risk management, automated investment analysis, process automation, and redefining customer services. Let us learn about a few of them in the next sections.
Risk Management
For a financial institution like a bank, risk management involves two major aspects - Identifying bad business opportunities and identifying fraudulent transactions. A business opportunity for a bank is a loan. Loans without adequate guarantee have a jigger interest rate while loans with property or automobiles to back it has a lower interest rate. Banks get higher profits when they provide loans without a guarantee. So they are always on the lookout for identifying customers who can pay. A seasoned bank officer will be able to identify this from the demographic of the customer and document evidence. A bank having historical data of all the loans it sanctioned and the profit it generated out of them can use it to build machine learning models. Anything from simple logistic regression to a deep learning classification network can be employed to do this with varying degrees of success and cost.
But a bank relying on its own data to do this has two challenges - It has to implement an elaborate data ecosystem to facilitate continuous data capture, modeling, and monitoring as below.
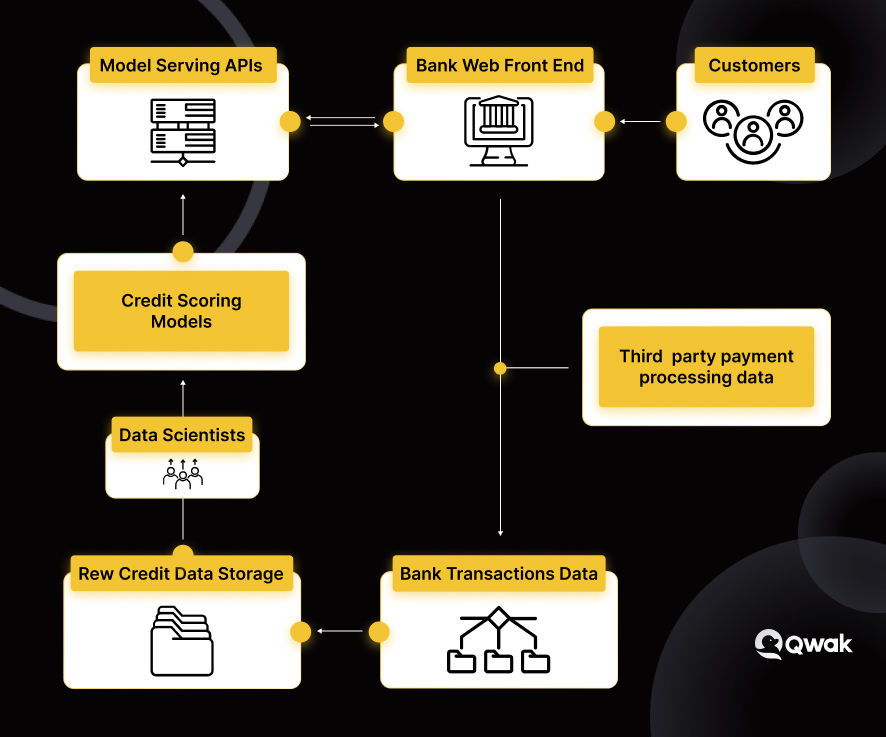
Even after this, the model will be based on data accumulated by that bank only. As we know machine learning models get better with more data. So what are the options for a smaller bank that is just starting its journey and does not have the budget for setting up a large infrastructure or enough historic data to rely on? It can rely on machine learning startups that provide credit scoring as a service. Zest.AI is one such startup that specializes in credit scoring.
Another aspect of machine learning in risk management is identifying fraudulent transactions. An expert human being can identify a fraudulent transaction just by looking at the account statements. For example, a person who has not spent a dime on alcoholic beverages has a transaction at a pub. An automated system based on rules can flag up this transaction and ask for an extra identity verification like an OTP. But doing this based on rules is not practical. This is where machine learning can help. Models can be trained on transaction patterns and can flag transactions in real time. The inference input to such a model will be a person’s profile embedding and transaction detail. HAWK.AI is one such startup that specializes in AI-based surveillance. It provides a service that can complement the rules with explainable AI for transaction monitoring and customer risk rating.
Automated trading and investments
Stock trading is a complicated business that requires deep knowledge of the fundamentals of stocks as well as behavioral aspects of the stock market. The stock price of a company with questionable fundamentals can go up only based on the hype generated by news. Companies with rock-solid fundamentals can stay as hidden gems because nobody bothered to analyze them. Machine learning can make a big difference here. Stock market data and news data are publicly available. Many stock brokers expose buy and sell features as APIs. All one needs to be successful in the stock market is an automated system that can analyze all the accumulated information and place automated buy and sell orders. But this is easier said than done and automated trading remains a holy grail now.
The amount of information that a model needs to process and the weightage that it should place on each factor is difficult to compute in the case of public markets. Startups like Tickeron and TrendSpider provide AI-based forecasting features that traders can rely on. They provide entry and exit prices with confidence intervals for different strategies. Some of them also provide pattern recognition engines that can watch out for price movement patterns defined by the user.
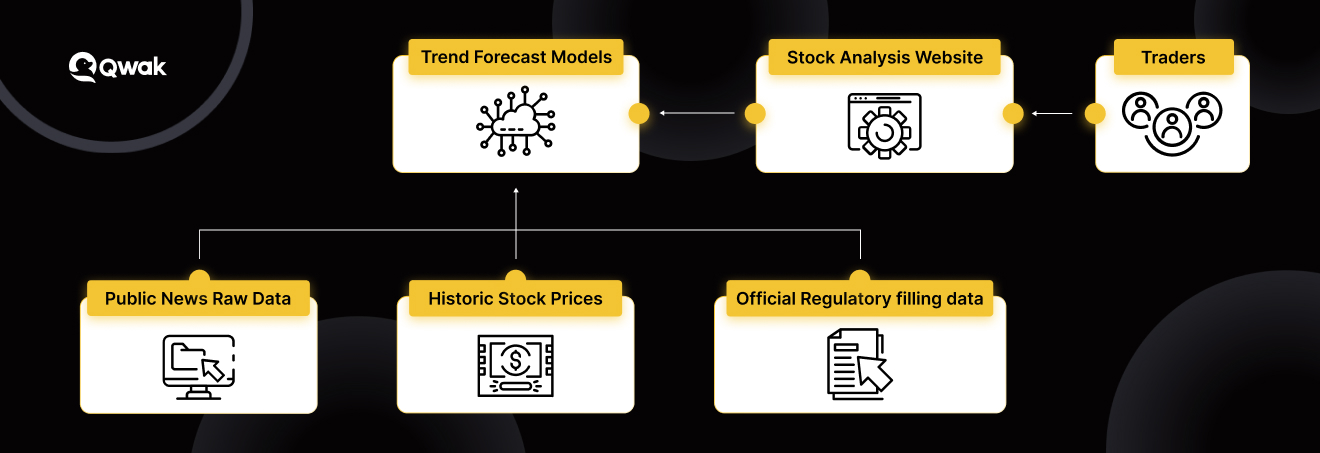
Investments are not only about identifying the asset that can provide you with the best benefit with the least risk. Such an elusive investment may not exist. So investment managers create a portfolio that contains risky investments with higher returns and low-risk investments with lesser returns. A lot of analysis and mathematics goes behind this process which optimizes a portfolio for defined risk and reward ratio. This is also an area where machine learning can make a difference. Startups like Axyon AI bring AI to investment management and optimizing portfolios based on asset classes.
Private equity investments require a different type of analysis compared to public stock trading. Investment banks specialize in identifying private equity investment opportunities and facilitating initial public offerings for private companies. Private equity investments rely on the fundamentals of the business and the ability of the promoter to run the business. The amount of data in the private equity world regarding historic good and bad investments is not great. The possibility of an automated model is less here. But machine learning models can replace human effort in analyzing millions of documents and account statements that private companies provide scores regarding data quality and authenticity.
Process optimization and automation
Banking organizations execute numerous manual back-office processes while dealing with customers. The account opening process includes a number of steps including identity verification, supporting document verification, referrer verification, etc. A business account opening process is more complex with even more documents to process and regulatory requirements. Banks and financial organizations can use pluggable machine learning services to automate many of these back-office processes.
For example, take the case of identifying document verification. Generally, a bank officer goes through an identity document like a birth certificate, or driving license and matches it against the person’s face to ensure authenticity. Image recognition and verification is an area where machine learning has made considerable progress in the recent past. There are many startups that focus specifically on identity verification and KYC facilitation. Onfido is one such company that aims to make identity verification simple through biometrics and machine learning.
Data entry is another area where manual effort is employed in back office operations. An employee reads through the documents submitted by customers and manually enters the details. Robotic process automation can help here. With current technology, applications that can parse specific documents like Passport, Driving License, etc, and extract relevant information from it are available. ML-based applications can also help in processing handwritten forms. Organizations like Mantl specialize in improving customer account opening rates by automating more than 90 percent of the tasks involved.
Forecasting revenue is another back office operation that is relevant to all organizations including financial organizations. Machine learning can help in forecasting cash availability and flagging abnormalities in accounting. It can also help in autonomous accounting and financial close management. Close management is a management account process where accountants verify and adjust account contents for a specific period like a quarter or year. Highradius is a company that specializes in AI-based autonomous treasury and accounting.
Customer services
Improving is not only about quicker decisions but also about minimizing the need for physical presence and personalized offerings. Even with the availability of self-service websites and mobile apps, many customers still choose to go to a physical location in many cases. Customers often struggle to find what they are looking for in a website or mobile application. A chatbot can reduce such issues by allowing customers to type in natural language about their requests and directing them to relevant sections. Chatbot frameworks like Azure Bots and Google Dialogflow provide tools to implement such chatbots. But they require considerable engineering effort and historic data to rise to their full potential. An architecture for implementing such a chatbot is shown below.
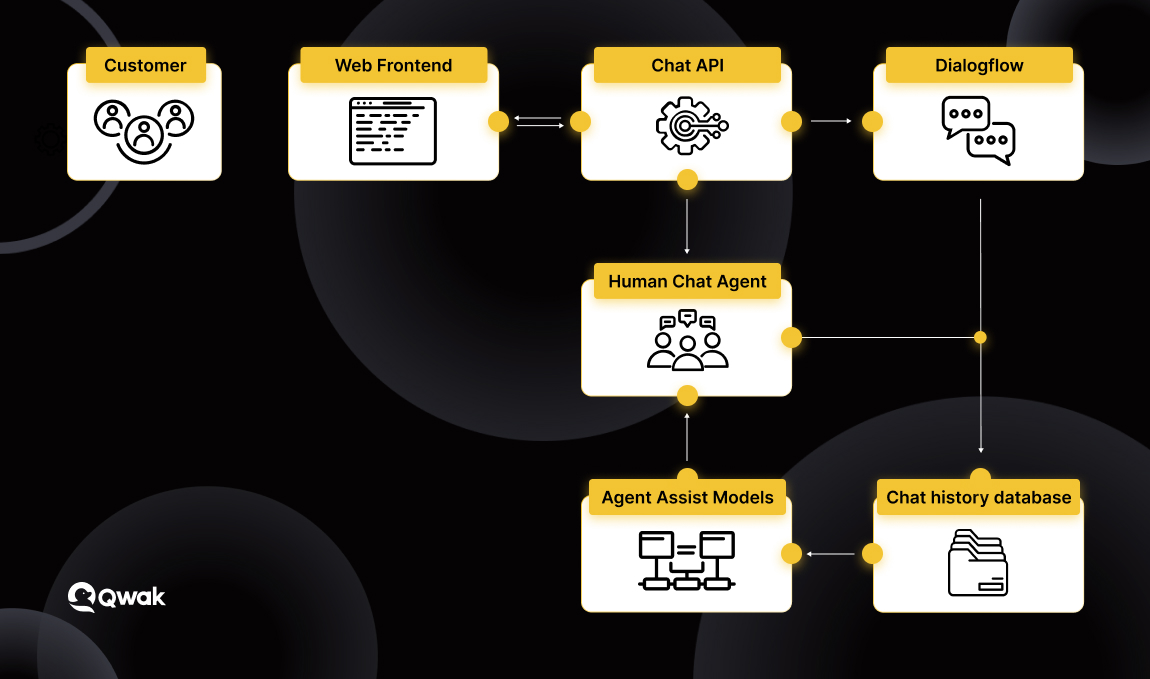
Smaller institutions and banks cannot afford to implement such elaborate architectures. Startups like Streebo provide chatbot services tailor-made for banking allowing financial institutions to focus on their core area and not worry about the IT engineering behind implementing chatbots. Active.AI is another such company specializing in conversational AI in the banking domain.
Offering personalized services helps banks to retain customers and sell more products. Machine learning can help here by learning customer preferences and developing product specifications that will appeal to the customer. BankBuddy provides cognitive services to bring personalized and frictionless banking across multiple channels.
Conclusion
Machine learning can make a big difference in the financial world by providing better risk management and customer service. It can also help in implementing automated investment strategies and accelerating the back office processes.
Having access to a well-designed machine learning platform can abstract away most of the work involved in solving these uncertainties. Such platforms allow developers to focus on the core problem without worrying about the complexities of deployment. Qwak is a machine learning platform that can streamline your complete model development and deployment process. You can check out Qwak here.